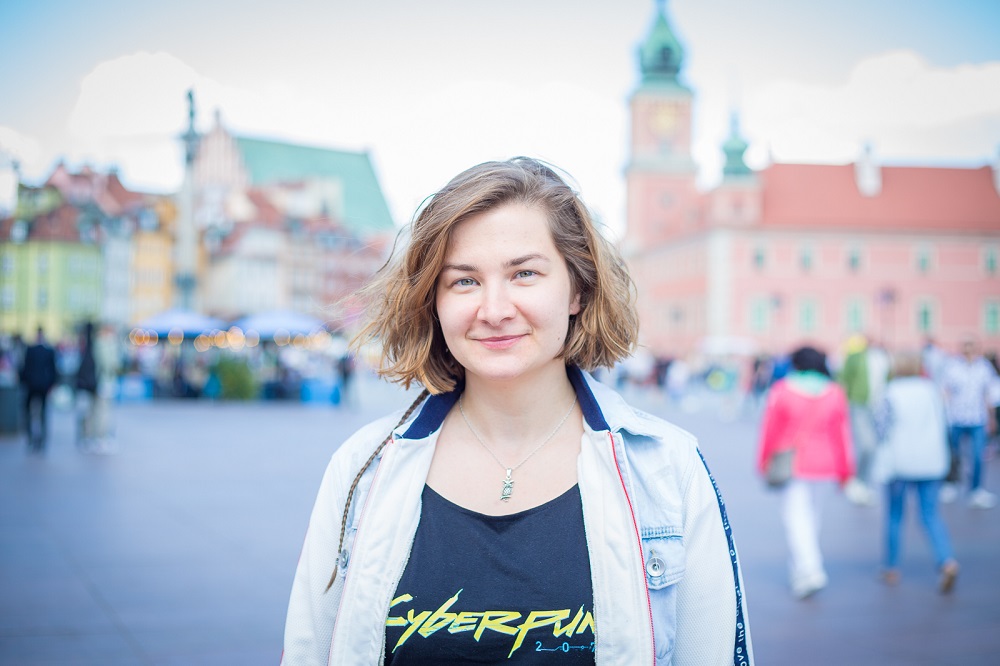
Photo by Ola Sierant
Castle Square, Warsaw
Dr Anna Dawid
Quantum physics & machine learning scientist,
theatre and games enthusiast.
Hi! I’m an assistant professor at the Leiden Institute of Advanced Computer Science (LIACS) at Leiden University in the Netherlands and a principal investigator in the aQa group. I am happily playing with interpretable machine learning for science, ultracold platforms for quantum simulations, and theory of machine learning. My scientific passion is molding automated approaches into a unique new scientific lens and looking through it at established difficult quantum problems.
Sounds interesting? I’m looking for a postdoc! 🙂
Before joining aQa, I was a research fellow at the Center of Computational Quantum Physics of the Flatiron Institute in New York. In 2022, I defended my joint Ph.D. in physics and photonics under the supervision of Prof. Michał Tomza (Faculty of Physics, University of Warsaw, Poland) and Prof. Maciej Lewenstein (ICFO – The Institute of Photonic Sciences, Spain). Before that, I did my MSc in quantum chemistry and BSc in biotechnology at the University of Warsaw.
I am also a 2022 FNP START laureate and one of the selected participants in the 2024 Lindau Nobel Laureate Meeting. On the second occasion, I answered “10 Questions” for the Women in Research Blog.
Research directions
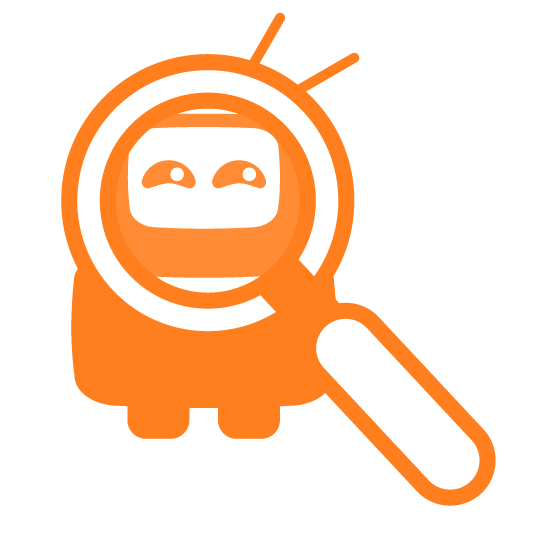
Understanding machine learning
Why overparametrized models generalize so well? Is generalization related to the flatness of the training loss minimum? What is the reason for double descent? What data features are learned by machines?
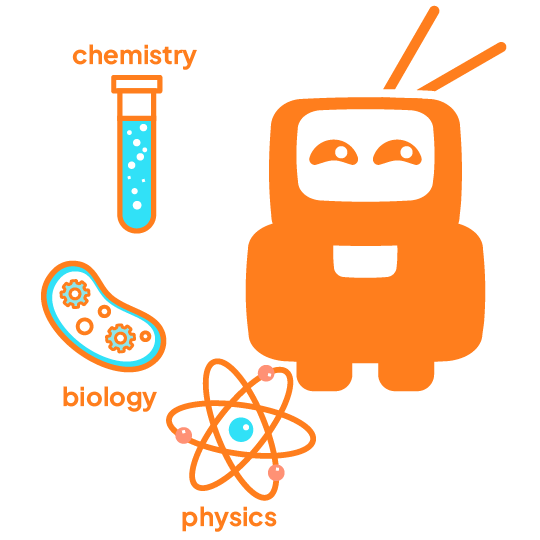
Machine learning for sciences
How to boost quantum experiments with deep learning? How to automatically detect local and global order parameters of quantum phase transitions? Can we learn new physics from trained neural networks?
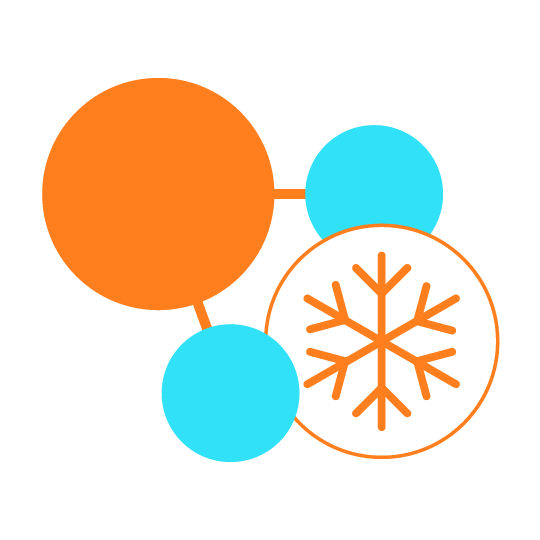
Ultracold molecules
What happens when we have both magnetic and electric excitations which are additionally coupled? Can we go beyond diatomic molecules with diagonal Franck-Condon factors with laser cooling?
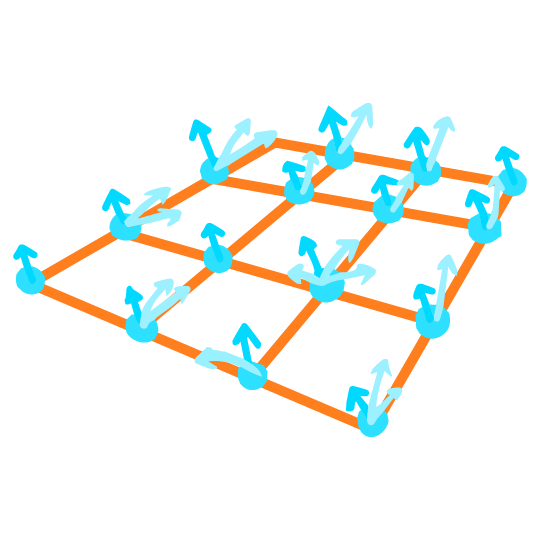
Quantum simulations
What novel phases of matter can we design? How to understand high-temperature superconductivity with quantum simulators?
Featured publications
O. Simard*, A. Dawid*, J. Tindall, M. Ferrero, A. M. Sengupta, A. Georges. Learning interactions between Rydberg atoms. arXiv:2412.12019.
A. Vysogorets, A. Dawid, J. Kempe. Deconstructing the Goldilocks zone of neural network initialization. ICML ’24, arXiv:2402.03579.
See the summary on X!
A. Dawid, N. Bigagli, D. W. Savin, S. Will. Automated graph-based detection of quantum control schemes: Application to molecular laser cooling. Phys. Rev. Research 7, 013135 (2025).
See the summary on X!
News
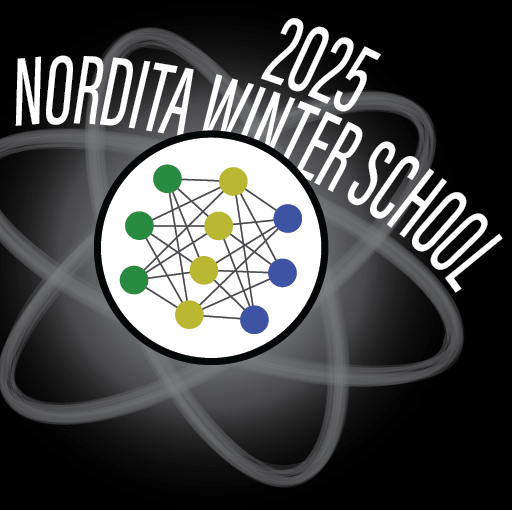
Jan 24, 2025
I’m back from my first ever school that I attended as a lecturer! It was Nordita Winter School on ML <-> physics. You can check out my lectures and tutorials on neural network loss landscapes and interpretability here 🙂
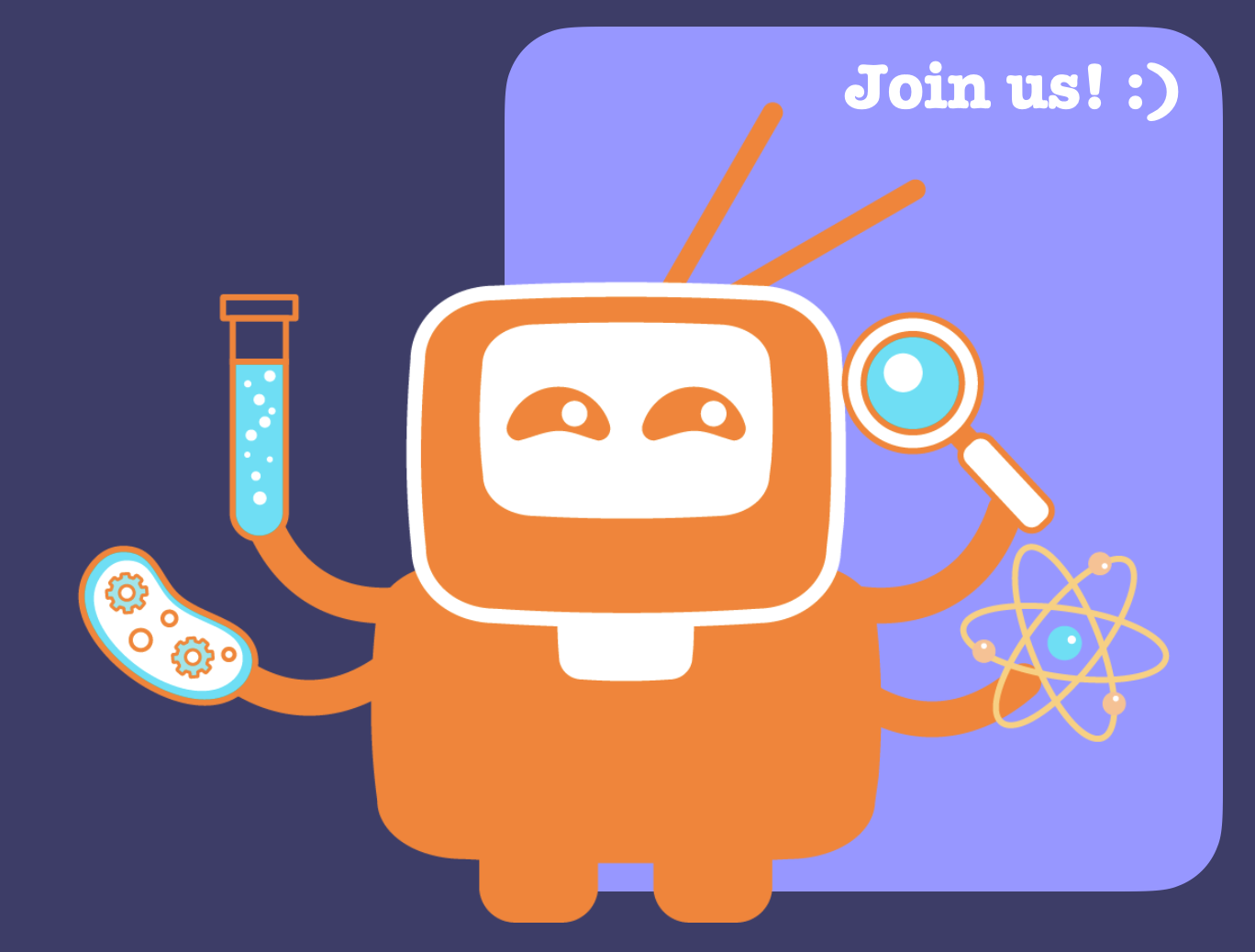
Oct 21, 2024
It is time to grow a group! If you are curious about interpretable machine learning for quantum problems, consider applying for a PhD student or a postdoctoral position! 🙂
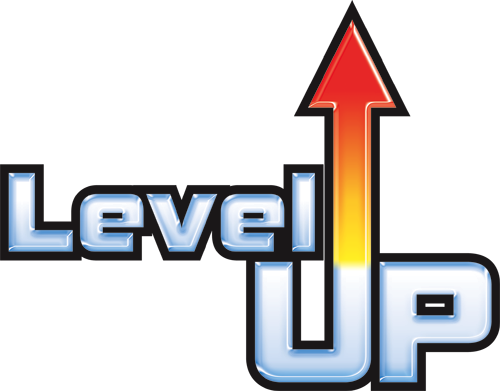
Aug 14, 2024
Level up! Starting this October, I’m kicking off a research group within aQa at Leiden University in Netherlands as an assistant professor! Just signed my soul away, I mean, a contract. I’m mostly excited and a bit frantic. Also, I try to forget that society has some expectations towards professors, but my friends tease me terribly about it 😛